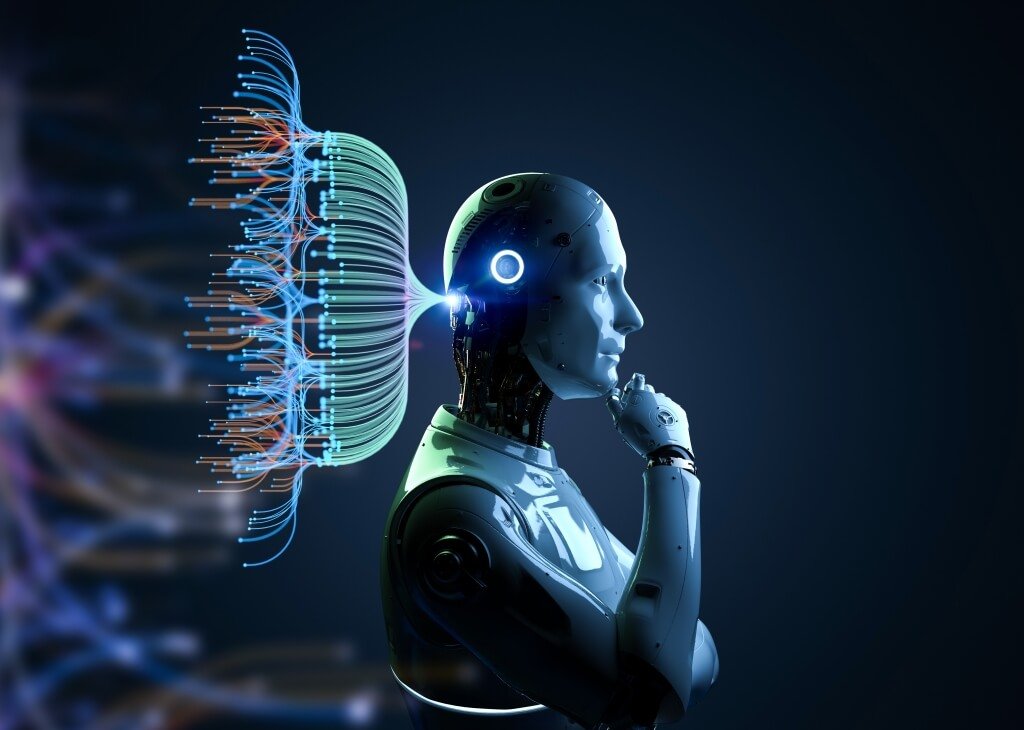
Unlocking the Power of Machine Learning: How Neural Networks are Changing the Game
Machine learning is a subfield of artificial intelligence that uses statistical techniques to enable computers to learn from data and make predictions or decisions without being explicitly programmed. Machine learning has become increasingly powerful in recent years, with the rise of big data, faster computers, and improved algorithms.
Machine learning is rapidly transforming various industries and sectors, including healthcare, finance, retail, and more. One of the key drivers of this transformation is neural networks, which are increasingly being used to solve complex problems that were previously unsolvable or difficult to solve using traditional methods. In this article, we will discuss how neural networks are changing the game in the field of machine learning.
What are Neural Networks?
The neural network algorithm is based on the structure and function of the brain. Like the human brain, a neural network is composed of interconnected nodes, or neurons, that work together to process and analyse data. These networks can learn and adapt to new data inputs, allowing them to improve their accuracy and effectiveness over time.
Also, it enables to process of complex patterns and relationships in data that human analysts may not notice right off the bat. As they process more data, they enhance their ability to recognise patterns and make accurate predictions. This is one of the key advantages of neural networks.
Neural networks are especially helpful in fields such as speech and image recognition, natural language processing, and even gameplay. The second key advantage of neural networks is their ability to learn from experience. Unlike traditional algorithms, which require explicit instructions, neural networks can make predictions and decisions based on massive amounts of data.
Neural networks benefits in the healthcare sector
One of the most exciting applications of neural networks is in the field of healthcare. Researchers are using these networks to develop new diagnostic tools and treatment plans for a wide range of conditions, from cancer to neurological disorders.
Here are some of the ways neural networks are being used in the healthcare sector, and how they are benefiting patients, providers, and the industry as a whole.
Predictive Analytics
One of the most significant benefits of neural networks in healthcare is their ability to perform predictive analytics. Neural networks can analyze vast amounts of data and use it to identify patterns, trends, and potential health risks. This can help healthcare providers identify patients who may be at risk of developing specific conditions, allowing for early intervention and better outcomes.
For example, neural networks can analyze data from a patient’s medical history, lifestyle, and genetic information to predict the likelihood of developing a particular disease, such as diabetes or heart disease. Providers can then use this information to create personalized treatment plans and preventive measures for at-risk patients.
Medical Imaging
Another area where neural networks are making a significant impact in healthcare is medical imaging. Neural networks can analyze medical images, such as X-rays, CT scans, and MRIs, and identify potential abnormalities, such as tumours or other anomalies, with greater accuracy and speed than human experts.
This can lead to faster diagnoses and more effective treatments, as providers can detect and address potential health issues earlier. Neural networks can also help reduce the number of false positives and false negatives, which can lead to unnecessary treatments or missed diagnoses.
Drug Discovery
Neural networks are also being used in drug discovery, where they can analyze large datasets to identify potential new drugs and treatments. By analyzing vast amounts of data, including genetic information and disease patterns, neural networks can identify new drug candidates and predict their efficacy.
This can help reduce the time and cost involved in drug discovery and development, potentially leading to faster and more effective treatments for patients.
Electronic Health Records
Electronic health records are a critical tool in modern healthcare, but they can also be complex and time-consuming to manage. Neural networks can help streamline EHR management by analyzing data from multiple sources and creating personalized treatment plans for patients.
Neural networks can also help healthcare providers identify potential errors or inconsistencies in patient records, leading to improved accuracy and better patient outcomes. Additionally, by analyzing patient data from multiple sources, neural networks can help identify potential drug interactions and adverse events, leading to better patient safety.
Personalized Medicine
Personalized medicine is an emerging field that aims to provide tailored treatments for individual patients based on their unique genetic makeup, lifestyle, and medical history. Neural networks are a critical tool in personalized medicine, as they can analyze large amounts of data to identify potential treatments and predict their efficacy for specific patients.
By using neural networks to analyze patient data, healthcare providers can create personalized treatment plans that are more effective and have fewer side effects. This can lead to improved patient outcomes and better quality of life for patients.
Neural networks benefits in the finance sector
In finance, neural networks are being used to analyze market data and make predictions about future trends. For example, a neural network can be trained to identify patterns in stock prices or other financial indicators that might indicate a forthcoming market crash or other major events. Traders and investors can use this information to make better decisions about asset purchases and sales.
Neural networks are used in self-driving cars
Neural networks are a critical component of self-driving cars, enabling the vehicle to perceive its environment and make decisions based on that perception. Specifically, convolutional neural networks (CNNs) are used for object detection and recognition, while recurrent neural networks (RNNs) are used for predicting future actions and behaviours of both the vehicle and other objects on the road.
In addition to self-driving cars, neural networks are also used in other transportation applications, such as traffic prediction, route optimization, and even air traffic control. By analyzing vast amounts of data, neural networks can identify patterns and make predictions that can improve the efficiency, safety, and overall performance of transportation systems.
Neural networks: Challenges being faced
One of the challenges of working with neural networks is that they require large amounts of data to train effectively. This data needs to be labelled and organized in a way that allows the network to identify patterns and relationships. Additionally, neural networks can be difficult to interpret, making understanding how they arrive at their predictions and decisions challenging.
Despite these challenges, the potential benefits of using neural networks are enormous. By unlocking the power of machine learning, organizations can gain new insights into complex problems, make more accurate predictions, and ultimately drive greater value for their customers and stakeholders.
How Neural networks are used in Retail industries?
Neural networks, a type of artificial intelligence model, can be used in various ways in the retail industry to improve various aspects of retail operations.
Here are some examples:
Customer segmentation and targeting
Retailers can use neural networks to segment their customer base and target specific groups with personalized marketing campaigns. By analyzing customer data such as purchasing history, demographics, and behaviour, retailers can identify patterns and trends and tailor their marketing efforts accordingly.
Fraud detection
Neural networks can be used to identify fraudulent activities, such as credit card fraud, by analyzing patterns in transaction data and flagging suspicious behaviour.
Price optimization
Retailers can use neural networks to optimize prices for products based on factors such as customer demand, competition, and pricing history. Profitability and sales can be increased as a result.
Inventory management
Neural networks can be used to optimize inventory levels by analyzing sales data and predicting future demand. This can help retailers avoid stockouts and reduce inventory carrying costs.
Customer service
Retailers can use neural networks to improve customer service by analyzing customer feedback and sentiment. This information can be used to identify common issues and improve customer support processes.
Demand forecasting
Retailers use neural networks to predict future demand for products based on historical data, seasonal trends, and other factors. This information is used to optimize inventory levels, reduce waste, and improve the accuracy of orders.
Conclusion
The rise of neural networks has been a game-changer for the field of machine learning. These algorithms have the potential to transform a wide range of industries by unlocking the power of data and opening up new possibilities for data analysis and decision-making.
As the technology continues to evolve and improve, we can expect to see even more exciting applications of neural networks in the years ahead.
Leave a Reply